The Power of Auto-Encoders in Training Large Healthcare Data.
The Power of Auto-Encoders in Training Large Healthcare Data
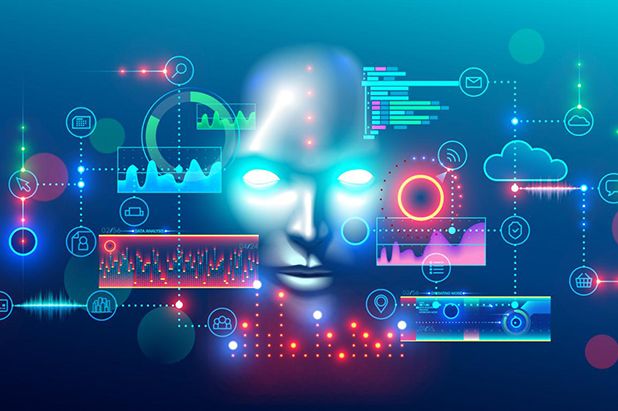
The healthcare industry is currently experiencing a data revolution, with vast amounts of information being generated from imaging studies, genetic sequencing, clinical records, and biomarkers. This influx of data presents both significant opportunities and challenges for healthcare professionals and researchers. One promising approach to managing and analyzing this complex data is the use of auto-encoders, a type of artificial neural network designed for unsupervised learning. Auto-encoders have demonstrated remarkable potential in efficiently processing and analyzing large-scale healthcare data, leading to advancements in diagnostics, personalized medicine, and disease prediction.
Understanding Auto-Encoders
Auto-encoders are neural networks that learn efficient representations of input data by compressing it into a lower-dimensional space (encoding) and then reconstructing it back to its original form (decoding). The primary goal is to minimize the difference between the input and the reconstructed output, capturing the most salient features of the data. This ability to reduce dimensionality and extract meaningful patterns makes auto-encoders particularly useful for handling complex and high-dimensional healthcare datasets.
Applications in Healthcare: Imaging Studies
Medical imaging, including echocardiograms, MRI, CT scans, and X-rays, generates vast amounts of data requiring sophisticated analysis techniques. Auto-encoders can enhance image quality, detect anomalies, and assist in segmenting regions of interest. For instance, they have been used to improve the resolution of low-quality images and remove noise, which is crucial for accurate diagnosis (Litjens et al., 2017). Additionally, auto-encoders can aid in identifying subtle patterns indicative of diseases, thus supporting radiologists in making more informed decisions.
Genetic Sequencing
Next-generation sequencing technologies have revolutionized genomics, producing massive datasets that demand efficient processing. Auto-encoders help compress genetic data while preserving essential information, facilitating downstream analyses such as variant calling and gene expression profiling (Zou et al., 2019). By learning compact representations of genomic sequences, auto-encoders enable researchers to uncover hidden genetic markers associated with diseases, paving the way for personalized treatment strategies.
Clinical Data
Electronic health records (EHRs) contain a wealth of information about patients' medical histories, treatments, and outcomes. However, the heterogeneity and sheer volume of EHR data pose significant challenges for analysis. Auto-encoders can integrate and harmonize diverse clinical datasets, extracting latent features that capture underlying patterns and trends (Miotto et al., 2016). These insights can inform predictive models for patient outcomes, risk stratification, and resource allocation in healthcare settings.
Biomarkers
Biomarkers play a critical role in diagnosing diseases, monitoring progression, and evaluating treatment responses. Auto-encoders can assist in identifying novel biomarkers by analyzing complex datasets derived from proteomics, metabolomics, and other omics technologies. By reducing dimensionality and highlighting key features, auto-encoders facilitate the discovery of biomarkers that may not be apparent through traditional statistical methods (Ching et al., 2018).
Challenges and Future Directions
Despite their potential, the application of auto-encoders in healthcare is not without challenges. Issues such as data privacy, model interpretability, and the need for large labeled datasets for validation remain significant hurdles. Moreover, integrating multi-modal data from different sources requires sophisticated architectures and algorithms.
Future research should focus on developing more robust and interpretable auto-encoder models tailored to specific healthcare applications. Collaborative efforts between data scientists, clinicians, and policymakers will be essential to address ethical concerns and ensure the responsible use of these technologies.
Conclusion
Auto-encoders represent a powerful tool for unlocking the potential of large healthcare datasets. By enabling efficient data compression and feature extraction, they offer valuable insights that can drive innovations in diagnostics, treatment, and disease prevention. As the field continues to evolve, auto-encoders are poised to play an increasingly pivotal role in transforming healthcare delivery and improving patient outcomes.